“A successful Predictive Maintenance (PdM) strategy focuses on driving valuable action that prevents unplanned downtime. In every organization, maintenance workflows are intricately linked to equipment conditions.
A computer maintenance management system (CMMS) cannot live siloed from an equipment condition monitoring system for too long. These systems must talk to each other to share valuable data and insights for a Predictive Maintenance Strategy to be effective.
Together these systems create a proactive, efficient, and future-ready organization.”
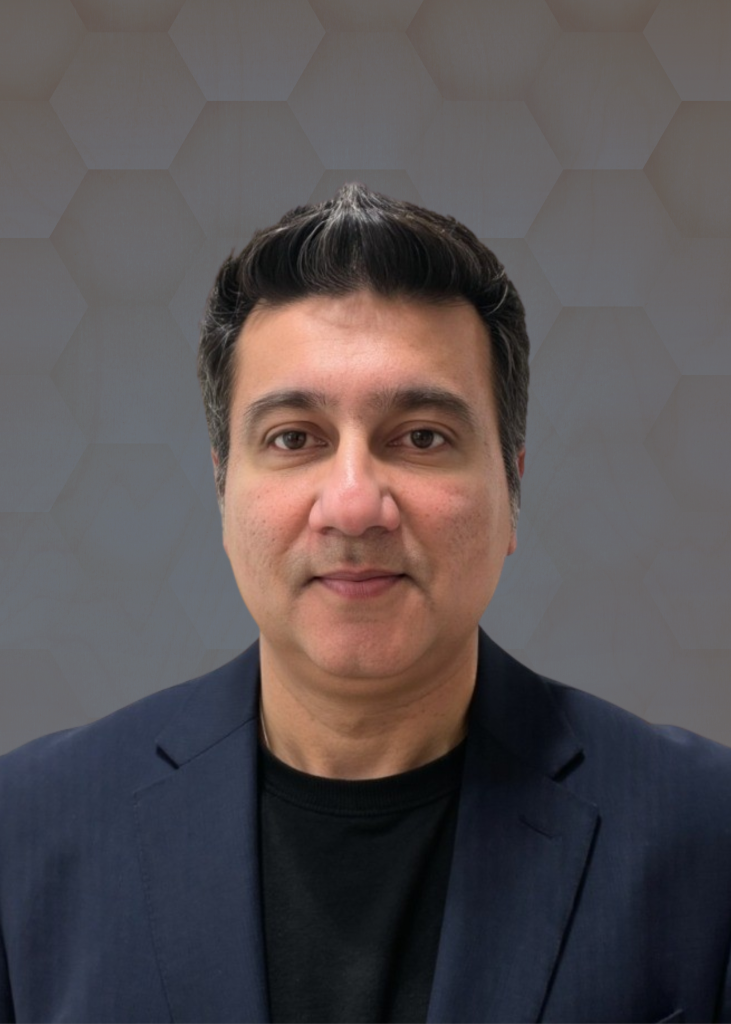
Chief Product Officer, Nanoprecise
Can I Get Real-Time Equipment Status in My CMMS?
That’s the question I hear most often when speaking with maintenance and reliability leaders at large manufacturing customers. The industry as a whole has made great strides – from handwritten logs and reactive responses to structured work orders and planned inspections. But even now, in highly connected and digitized plants, I still hear this:
“We have the data – but getting it into our CMMS and acting on it just at the right time is a struggle.”
This isn’t a technology limitation – it’s a systems’ architecture issue. 44% of facilities managers find work order tracking time-consuming, and 48% of delays in work execution stem from miscommunication – often due to a lack of visibility into real-time equipment condition. (Source)
Today’s industrial environments demand more than visibility – they demand connected, intelligent, responsive workflows. To deliver on that, we need to break down the barriers that continue to exist between the systems that detect failure and the systems that manage response.
The Role of CMMS and Why Real-Time Predictions Need Real-Time Workflows and Vice-versa
Computerized Maintenance Management Systems (CMMS) have become foundational to asset-intensive maintenance operations.
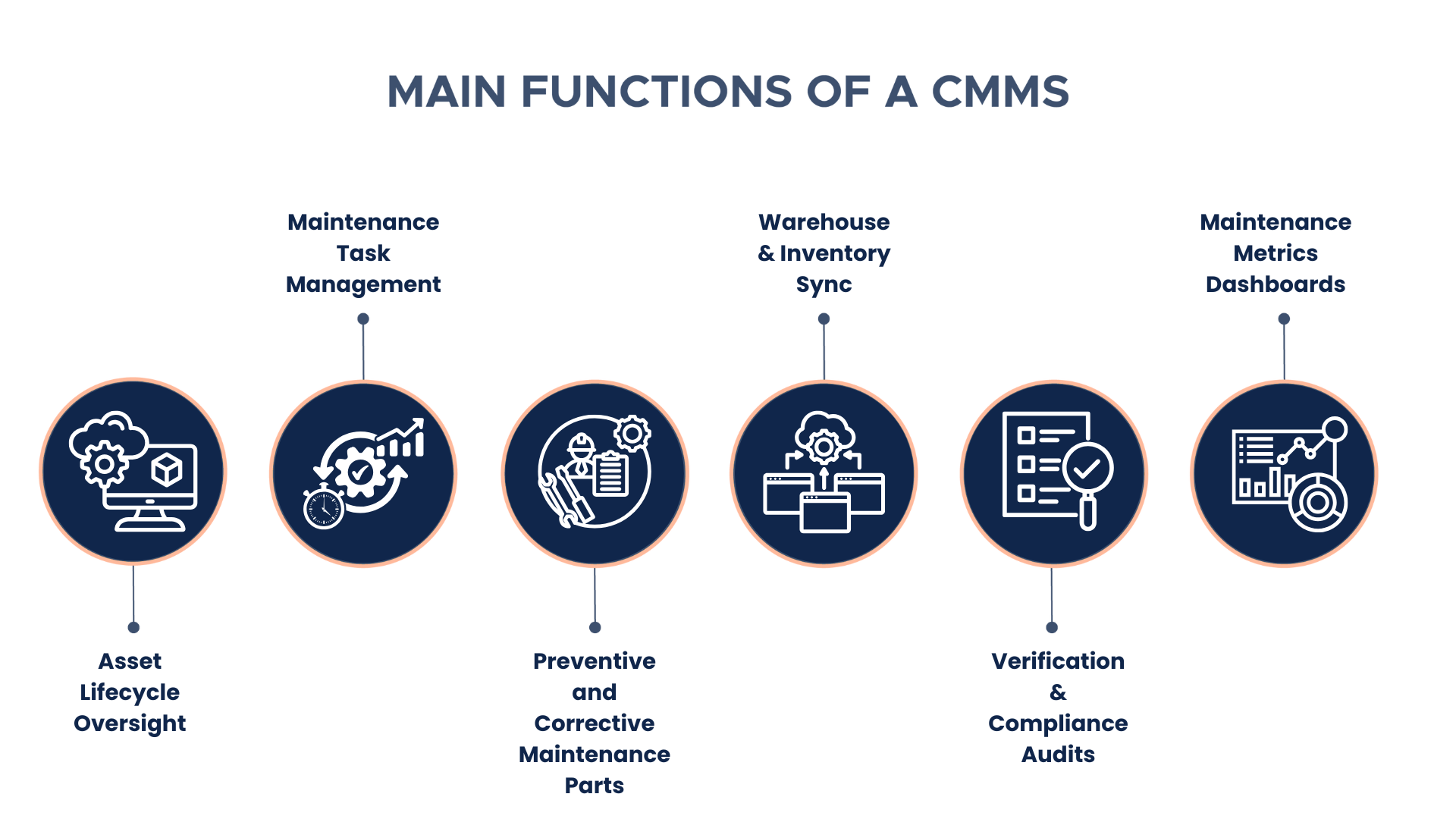
There’s no debate – the CMMS is a very powerful tool.
But it was never designed to predict failures, nor was it designed to process high-frequency equipment monitoring data. It’s a system built to manage action, not to diagnose equipment health. And that’s where the opportunity lies: to complement, and augment.
The Reality Beyond Work Orders: The Case for Real-Time Maintenance Intelligence
As maintenance strategies mature, organizations are moving from being reactive to being predictive and further to being prescriptive. But here’s the challenge – no matter how well one implements their CMMS, if it isn’t integrated with real-time equipment condition data and insights, it’s always a few steps behind.
Don’t Ask a CMMS to Predict Failures
It is not a system designed to sense the shifts in vibrations or temperature that may signal early-stage equipment failures. To make matters worse, only 48% of CMMS users have even implemented a predictive maintenance strategy. That leaves more than half of the maintenance teams in the world relying on semi-automated schedules and hope. (Source)
Put Humans in the Loop Where It Matters Most
CMMS platforms do an excellent job of automating work order flows. But when condition data from vibration analysts, data analysts, sensors, or external dashboards isn’t natively connected, the maintenance team is forced into a human-as-integrator role – manually interpreting data and insights, transferring these into the workflow for relevant actions. That’s how most organization operate and It’s a reflection of a strategy that underestimates the complexity of real-time decision-making.
What’s needed is a loop where:
- AI detects the fault, suggest a prescriptive action.
- The CMMS initiates the right action via a workflow.
- AI keeps the workflow up-to-date with real time fault criticality and escalations.
- And the human expert steps in where judgment is needed most (in the case of escalations).
- The human expert then re-educates the AI when required.
This is about placing human experts at the highest point of leverage.
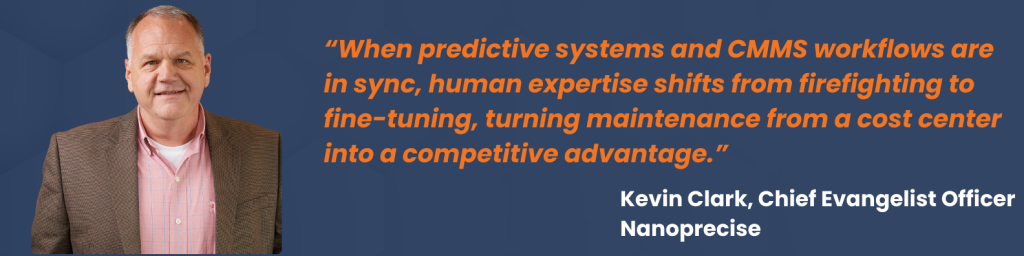
Don’t just Prioritize Maintenance for Downtime
While downtime remains a critical driver for production assets, it’s not the only lever maintenance teams must consider. A Condition Intelligent technology allows customers to prioritize:
- Downtime-risk for production-critical assets.
- Energy-inefficiency in high-consumption and/or low-criticality equipment.
- Emerging faults in non-instrumented assets that may otherwise be ignored.
This allows for a tiered financially optimized maintenance strategy that maximizes value – not just availability.
Eliminate The Real Problem – Barriers Created by The Siloed Strategy
Let’s be clear: a CMMS should not operate in isolation by design. Things become siloed when the broader maintenance strategy treats condition monitoring, planning, and execution as separate, sequential steps rather than be parts of a unified system. When our condition data and maintenance workflows exist in separate systems – with no feedback loop, no shared context, and no prioritization logic – we miss the chance to act intelligently
Agility Demands Integration: Predict – Plan – Act, Together
Organizations today aim to be agile – leveraging collaboration, continual learning, and incremental delivery. But agility in maintenance only happens when the system that detects equipment failure is tightly coupled with the system that responds to it.
In an ideal state, this coupling is bi-directional:
- Real-time notifications generate work orders automatically.
- Maintenance execution data flows back into the analytics engine to improve future insights.
This is where real-time data and analytics meets proactive execution.
This is where Condition Intelligence in an Energy-Centered Predictive Maintenance system and CMMS come together to power “AIM” — adaptive, intelligent maintenance.
To better understand this, let’s consider the P-F Curve, a graph used to identify asset reliability and performance over time. It illustrates that the earlier you detect a failure (the “P” point), the more time you have before functional failure (the “F” point). But if the CMMS isn’t aware of the “P” point, it can’t really do anything to prevent “F.”
Here’s the catch: detecting “P” is only half the equation. If there’s a manual bridge between your diagnostic system and your CMMS – like a technician reviewing alerts, transferring them into an email or spreadsheet, driving prioritization using a separate mechanism – that delay eats into your response window.
By the time a work order is created and prioritized:
- The asset may have already degraded further.
- The failure may be imminent or may have already occurred.
- Or the opportunity to optimize for energy efficiency may be lost.
Agility breaks down when workflows depend on any kind of middleware. To truly respond in time – and continuously improve – you need systems that:
- Detect failure accurately and early.
- Provide failure prevention recommendations.
- Automatically trigger the right workflow, and subsequent actions.
- Feed the outcome back into the detection layer.
Only then can you move from reactive firefighting to predictive, intelligent, iterative and efficient maintenance. (Image Source).
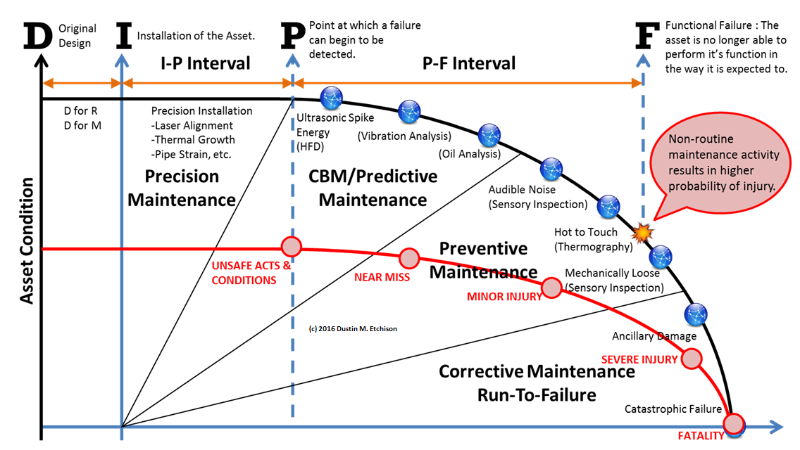
Field Observations: When Predictive Maintenance (PdM) Isn’t an Integrated Approach
While the value of predictive maintenance is well understood, we continue to observe a common pattern across industries: companies deploying PdM solutions that are either siloed from their CMMS or offer only partial integration.
Here’s what that looks like in practice:
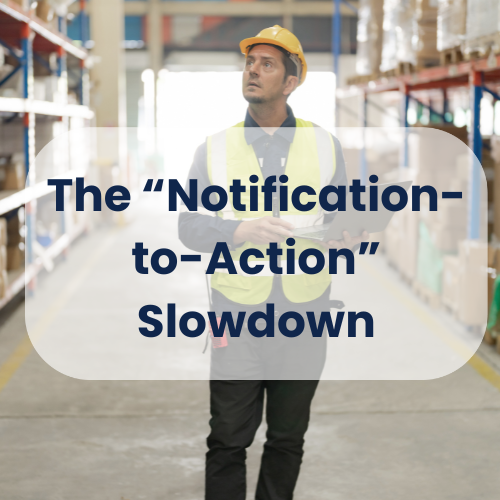
Insights from PdM systems are often delivered through dashboards or email notifications. But without seamless CMMS integration, the burden of interpreting these insights and creating work orders manually falls on individuals. This delays response time and increases the risk of preventable failures.
In the absence of integration, teams resort to workarounds like spreadsheets, offline prioritization boards, or ad hoc tools.
These methods introduce inefficiencies, increase the potential for errors, and become difficult to scale across large teams or sites.
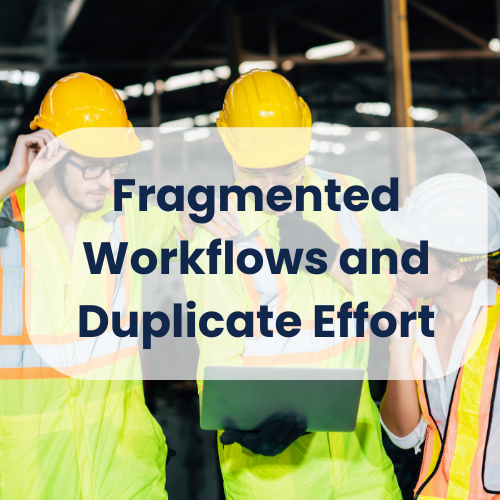
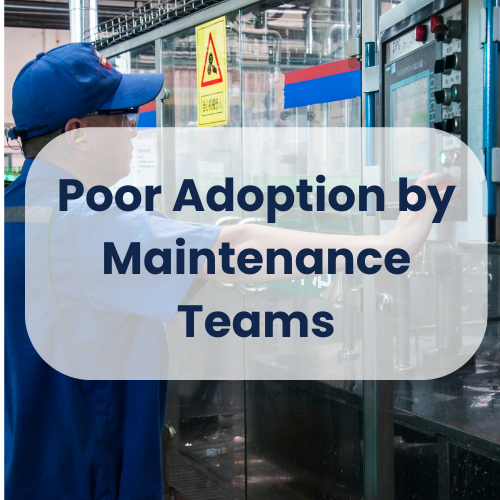
Most maintenance professionals rely on CMMS as their central operational tool.
When PdM insights aren’t delivered through the same system, they’re often overlooked—or mistrusted. This results in underutilization of the PdM platform and missed opportunities for proactive action.
Some PdM systems require complex custom integrations or work only with a limited set of CMMS platforms. This slows deployment, creates ongoing IT dependencies, and limits the flexibility enterprises need to standardize across multiple facilities.
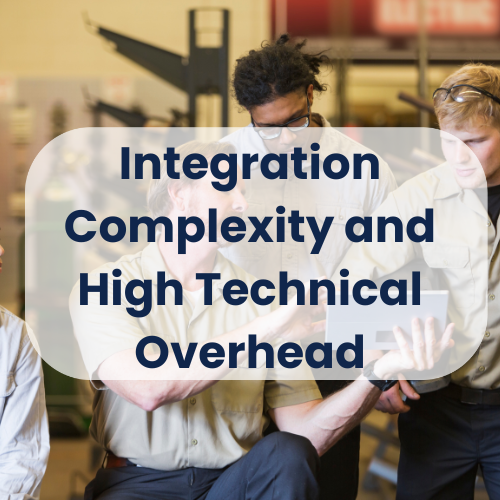
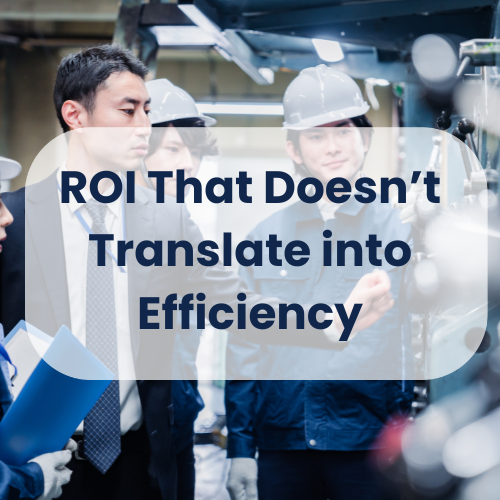
We’ve seen organizations invest significantly in predictive maintenance across their asset base, yet struggle to demonstrate ROI. The reason? While insights are generated, they don’t always make it to the systems or people responsible for acting on them. As a result, potential savings remain theoretical, not realized.
When PdM and CMMS operate in silos, the result isn’t just a technical gap, it becomes a strategic one. Disconnected tools lead to disconnected teams, disconnected workflows, and ultimately, disconnected operations. This misalignment makes it difficult to link maintenance actions with broader goals like energy efficiency, sustainability, or performance improvement.
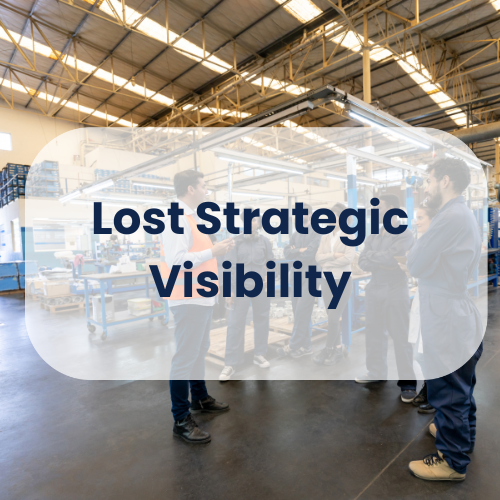
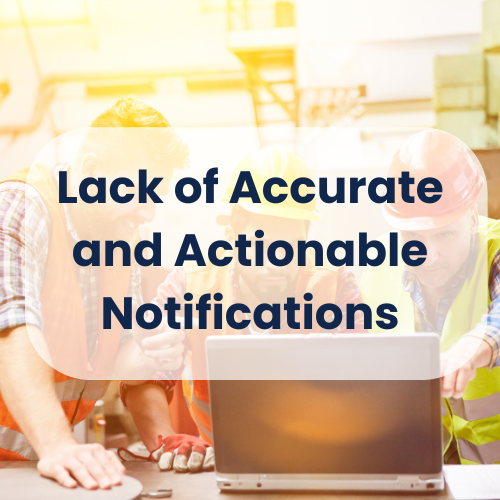
Many PdM tools can surface anomalies, but few provide the right level of context, criticality, and actionability. Maintenance teams are either overwhelmed with false positives or left guessing the next step. This creates noise instead of clarity and ultimately erodes trust in the system.
This is why Condition Intelligence within an Energy-Centered Predictive Maintenance Solution was built.
Nanoprecise’s Condition Intelligence in it’s ECM solution solves this by offering high-fidelity, CMMS-agnostic, integration-ready insights that are:
- Accurate enough to trust.
- Actionable enough to automate.
- Context-rich enough to prioritize.
Because great insights without structured execution are wasted. And predictive maintenance that lives in a dashboard, never driving action, leaves you still reacting, just slightly earlier.
With Condition Intelligence, predictive insights don’t just get noticed. They get routed, acted upon, and learned from seamlessly, across your existing CMMS ecosystem.
How It Works: Condition Intelligence That Can be Integrated into Your CMMS
At Nanoprecise, we’ve built an AI based critical equipment state detection engine that can accurately identify “correlated deviations” from normal operating equipment condition, utilize equipment state history, and leverage any other additional equipment data (type descriptions, manuals, etc.) to perform real-time predictive analysis. The real-time diagnostic results from this engine can easily be integrated with your CMMS – so that condition detection instantly connects to action. The figure below explains the typical working of a well integrated system.
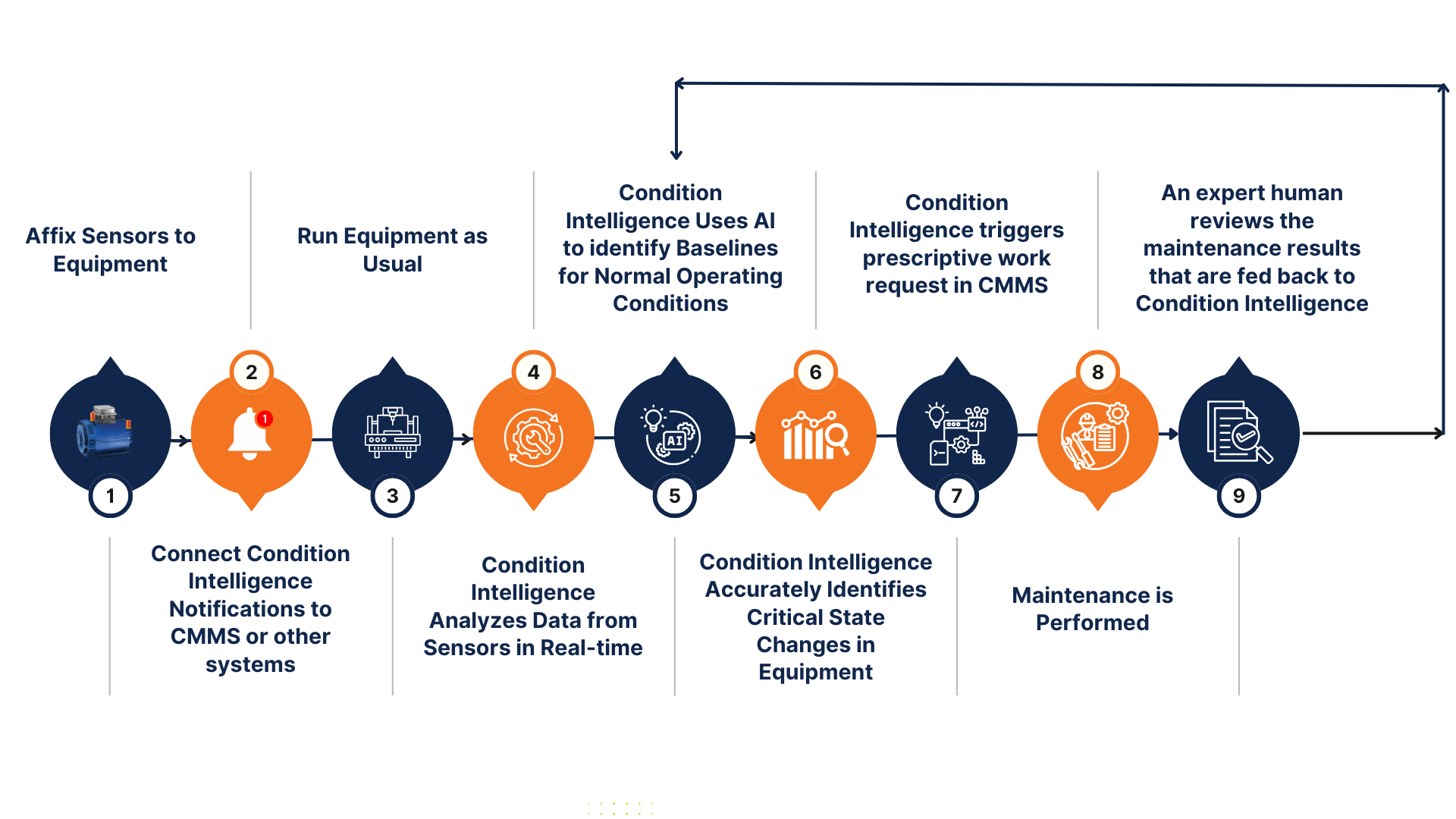
Why Should You Care?
In today’s fast-paced industrial world, efficiency is everything. Companies that fail to integrate predictive analytics with their CMMS are not just losing operational efficiency – they’re gradually falling behind the curve.
Your Competition Is Already Doing It
Organizations that have an integrated predictive maintenance strategy are seeing up to 30% fewer equipment failures and 15% longer asset lifespans. (Source)
Your Maintenance Budget Is at Risk
With rising energy prices, supply chain disruptions, and labor shortages, companies can’t afford inefficient workflows. Studies show that businesses with disconnected systems spend 25–40% more on repairs. (Source)
Your Sustainability Goals Depend on It
Energy waste from inefficient equipment contributes significantly to CO₂ emissions and costs. Companies optimizing maintenance through intelligent automation reduce energy usage by up to 15%. (Source)
The Cost of Inaction Is Higher Than You Think
If every day predictive insights aren’t connected to execution, inefficiencies compound. Avoidable downtime, redundant repairs, and missed savings add up fast.
Bottom Line: An integrated predictive maintenance strategy using a powerful Energy-Centered Predictive Maintenance solution coupled with your CMMS is a strategic business imperative.
So the real question isn’t “Why should you care?”, but “How long can you afford not to care?”
From Data to Dollars: Real-World Impact
- A biofuel plant prevented $18,000 in downtime losses by automating insight-to-action workflows.
- An oil & gas refinery detected a $625,000 failure in advance by auto-triggering prioritized maintenance.
- A leading chemical manufacturer in Spain avoided €50,000 in losses and saved €9,500 in energy by addressing faults early.
These results weren’t theoretical. They were the outcome of integrating a powerful ECM solution with Condition Intelligence directly into your execution systems.
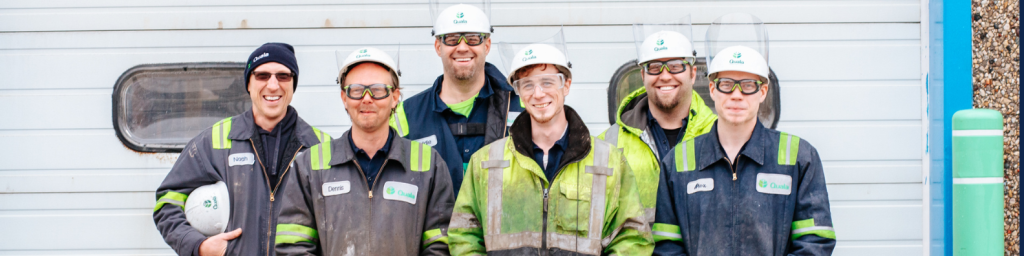
What’s Next? Industry 5.0. Predict. Prevent. Optimize.
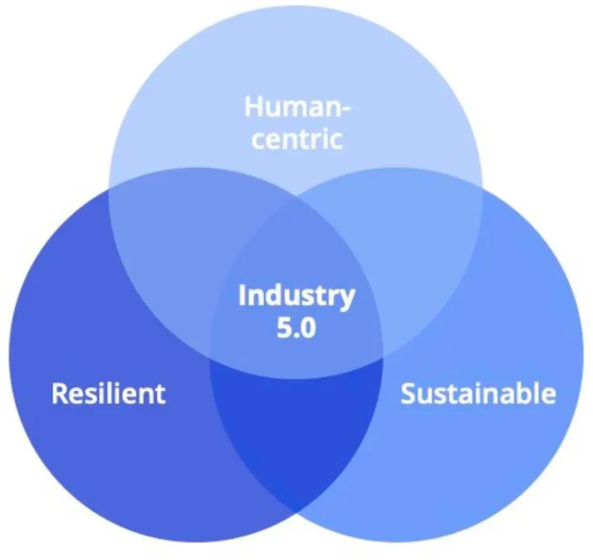
Industry 5.0 is graduating towards being human-centric, sustainable, and system-aware. Your maintenance strategy should be too.
Integrating Condition Intelligence into your CMMS is about unlocking their full potential.
In part 2 of this blog, we will get into the “HOW” part of integrating Condition Intelligence with leading Computerized Maintenance Management/Enterprise Asset Management systems. Watch this space for more innovative action from Nanoprecise product and engineering team.
Let’s connect your insights to action – seamlessly. Book a Demo Here
About the Author
Param Desai, CPO Nanoprecise
Param Desai thrives at the intersection of technology, strategy, and execution, transforming deep-tech vision into market-leading products. As Chief Product Officer at Nanoprecise Sci Corp, he drives product innovation, GTM execution, and customer success, ensuring Nanoprecise’s cutting-edge AI and predictive maintenance solutions deliver real-world impact.
Armed with 20+ years of rich industry experience, an Executive MBA from the University of Miami Herbert Business School and a Master’s in computer science from University of Florida, Param pairs deep technical expertise with strategic insight.
As a Stanford Executive Institute alum, he’s passionate about networking to build, scale, and redefine the future of deep-tech. you can connect with him on his LinkedIn.