A step-by-step guide to implementing predictive maintenance for reduced downtime, cost savings, and improved equipment reliability
Unplanned downtime can have devastating effects on productivity and return on investment. Predictive maintenance (PdM) helps prevent these disruptions by identifying potential failures before they occur.
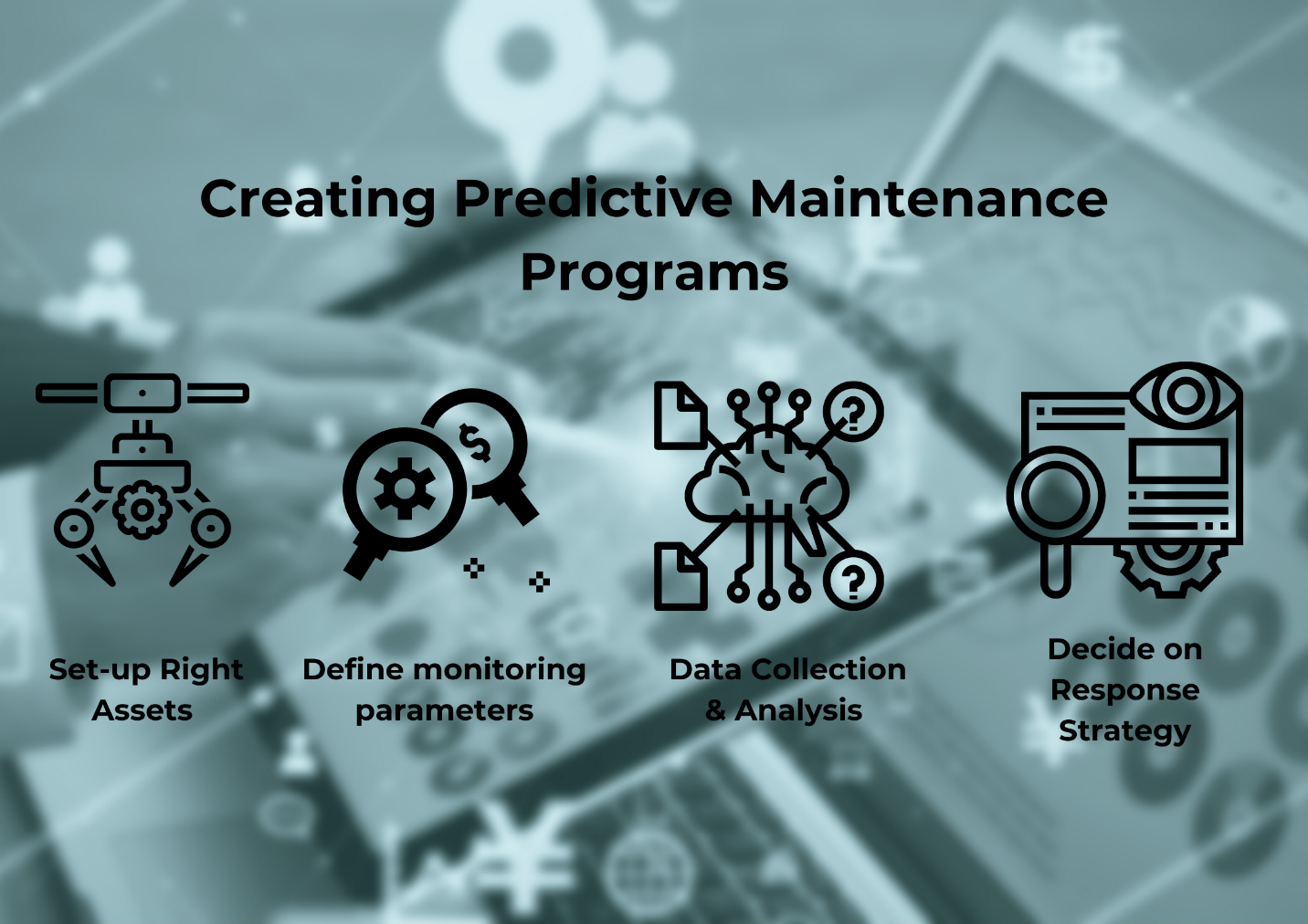
In order to establish a Preventive Maintenance Program, it is critical to establish a solid foundation, consisting of clearly defined methodology and desired outcomes. Organizations must adopt a robust strategy to successfully embrace predictive maintenance programs. Some basic steps towards achieving a successful implementation are:
- Establish a plan
- Choose right assets for PdM
- Select the right condition monitoring methods and equipment
- Establish Data Collection & Analysis mechanism
- Define a response procedure
- Build a data analysis strategy
- Establish a continuous implementation and improvement process
Let’s dive a little deeper into each of the steps, to understand how-to create an effective Predictive Maintenance Program:
- Establish a plan
Transitioning to predictive maintenance is a process and not a single event. Organizations must assess their current maintenance approach, define key goals, and align predictive maintenance efforts with overall business objectives. A well-structured plan considers:
- The scope of implementation
- Available resources and budget
- Integration with existing maintenance strategies
- The technological infrastructure needed for data collection and analysis
Laying a strong foundation ensures that predictive maintenance delivers measurable improvements in efficiency, cost savings, and equipment reliability.
- Choose right assets for PdM
The success of the predictive maintenance program depends upon the right selection of assets. Not all assets are suitable for predictive maintenance. Prioritizing the right equipment is essential for maximizing benefits. Ideal candidates for PdM include:
- Assets with a history of frequent failures
- Critical equipment that requires constant condition monitoring
- Machines prone to unexpected breakdowns that could halt production
- Equipment that is costly or difficult to replace
- Hard-to-reach or remote assets located in hazardous environments
- Select the right condition monitoring methods and equipment
Once the target assets are identified, the next step is selecting the appropriate monitoring techniques. These methods may include tracking parameters such as:
- Vibration – Useful for rotating machinery
- Temperature – Helps detect overheating issues
- Acoustic – Can reveal hidden faults in equipment
- RPM and other operational metrics – Essential for assessing performance
Choosing the correct parameters and monitoring tools is crucial, as they directly impact the accuracy and effectiveness of predictive maintenance.
- Establish Data Collection & Analysis mechanism
The next step is to monitor and collect data from the assets for establishing a baseline. This will help the algorithms evaluate the health of the machines and predict any anomalies. With enough data, the algorithms can learn the machine statistics to predict any future maintenance requirements and the time-till-failure for these machines / components. Cloud-based storage and advanced artificial intelligence systems that display results on a simplified dashboard, can help analyze vast amounts of machine-health data, and provide faster conclusions, thereby making it a simple and easy-to-use mechanism.
- Decide a response procedure
The next step in predictive maintenance is establishing a procedure to respond to anomalies. You may need a full-time technician who can monitor the statistics on the dashboard and communicate any anomalies / discrepancies in the health of the machines. Based on the results, they may give instructions to either shut down the system, run for a limited time or run indefinitely with constant monitoring.
- Build a data analysis strategy
A proper data analysis strategy must be established, especially for continuous monitoring. It is a crucial component of any PdM program. To maximize the benefits of predictive maintenance, companies must establish a strong data analysis strategy. This can be managed internally by trained experts or outsourced to third-party service providers. Online condition monitoring solutions allow remote access to data, enabling maintenance teams to track machine health and address issues proactively. For organizations with limited in-house resources, these systems provide a cost-effective alternative to hiring dedicated specialists.
- Establish a continuous implementation and improvement process
Predictive maintenance is not a one-time initiative, instead it requires ongoing optimization. You need to begin a cycle of implementation of predictive maintenance. You need to continuously evaluate your PdM program by measuring performance against key performance indicators (KPIs). To ensure the growth of the program, the PdM system must regularly monitor the data from critical assets. This needs proper understanding of the processes. From the experiences of past implementations, you can improve the predictive maintenance program to reduce maintenance costs, repair spending, unplanned downtime, and breakdowns.
Conclusion
In an increasingly competitive manufacturing landscape, predictive maintenance provides a significant advantage. It enhances productivity, reduces downtime, and helps organizations operate more efficiently.
By following a structured, data-driven approach, businesses can successfully implement predictive maintenance, ensuring long-term reliability and cost savings.